Warning signs for OpenAI and Google if the new AI model is not as smart as expected
OpenAI’s next big language model may not be as powerful as many hope
Dubbed Orion, this artificial intelligence (AI) model is working well behind the scenes, showing little progress compared to that of GPT-4 compared to GPT-3, Bloomberg reported.
According to Bloomberg, not only is OpenAI having difficulty with new AI models, but it is also not performing as well as expected.
These industry issues may be a sign that current AI model innovation is moving in a “run-through” direction.
“The AGI bubble is bursting a little bit,” Margaret Mitchell, chief behavioral scientist at startup Hugging Face, told Bloomberg, adding that “different training methods” require different levels of intelligence. intelligent and flexible like humans to overcome any problem.
The motto that has brought success to Generative AI to date is increasing: make a Generative AI model more powerful, the main way is to make it bigger.
But as these AI models get bigger and more powerful, they also become more power-hungry.
Technology companies are using computer-generated artificial intelligence to find new “brains” for AI.
New Enterprise Associates (NEA) is one of the largest and oldest venture capital firms in America.
According to Dario Amodei, CEO of Anthropic, it currently costs $100 million to build an advanced AI model and is estimated to cost more than $10 billion by 2027.
Has the golden age passed?
This year, Anthropologie updated the Claude models, but notably without the Opus, mentioning the model’s recent release date, which has since been removed from the company’s website.
Like OpenAI, anthropologists also see only modest improvements in Opus, despite its large scale and the cost of building and operating it, according to Bloomberg sources.
According to a Bloomberg report, Google’s Gemini is not achieving its goals.
Clearly, these are not insurmountable challenges.
“We have achieved rapid growth in a short period of time,” said Noah Giasirakos, assistant professor of mathematics at Bentley University in Massachusetts (USA).
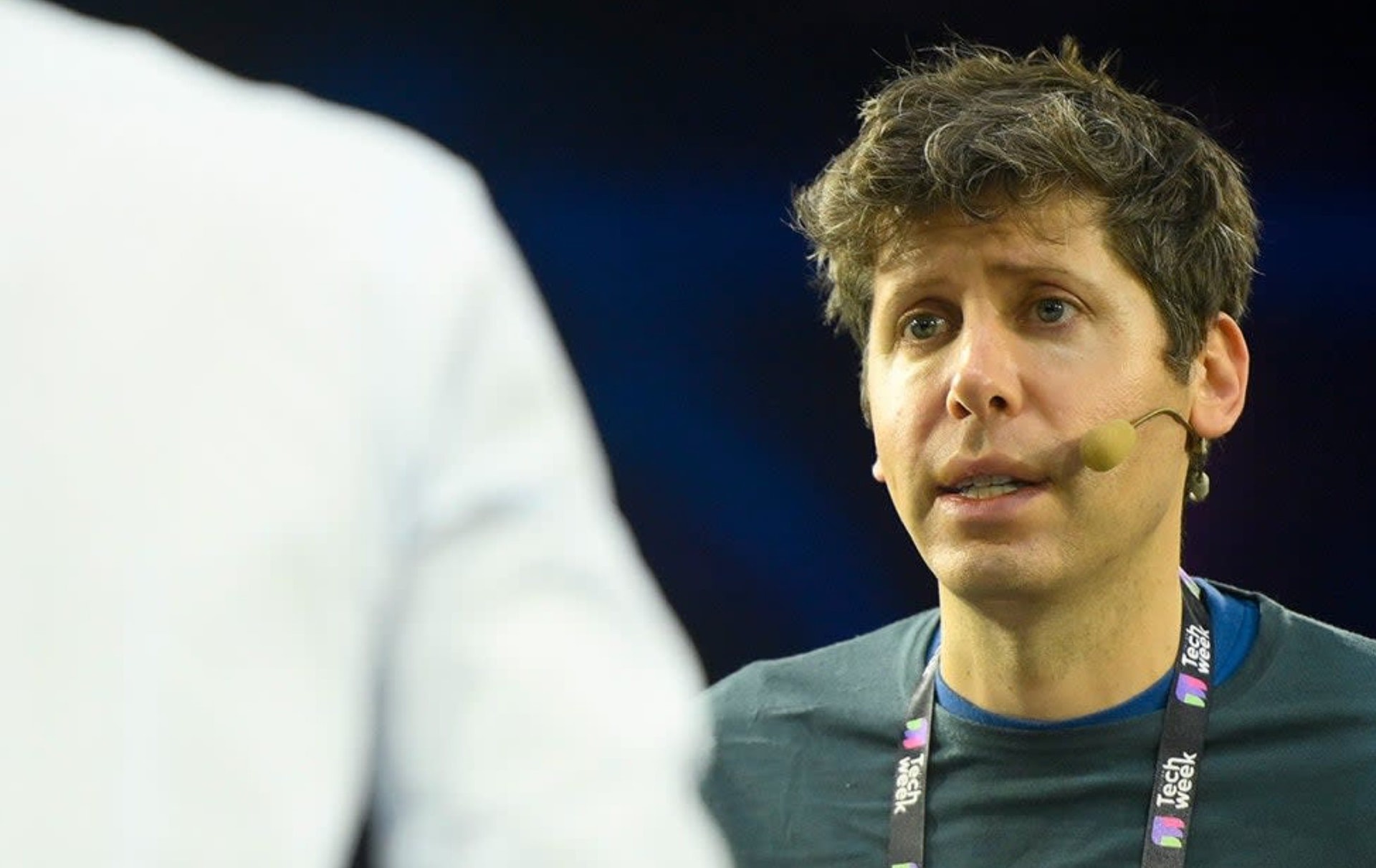
OpenAI and many companies are looking for new ways to make AI smarter where current methods are limited
OpenAI and other AI companies are always looking to overcome the unexpected delays and challenges in creating large language models by developing training techniques so algorithms can “guess” them. .
Dozens of scientists, researchers and AI investors told Reuters they believe these techniques, which underpin the o1 large language model recently released by OpenAI, could reshape the race. AI and influence the types of resources some companies require, from power to chips.
Since OpenAI Chat launched its GPT chatbot two years ago, tech companies (which benefited greatly from the AI craze) have been saying that ever-increasing data and computing power will lead to AI models. increasingly improved.
Ilya Sutskever, co-founder of OpenAI and Safe Superintelligence, recently told Reuters that scaling up pre-training (an AI model that uses large amounts of unlabeled data to understand language patterns and structures) ) has reached its limit.
Ilia Sutskever, former chief scientific officer of OpenAI, was an early advocate of making major advances in the field of general AI by leveraging large amounts of data and computing power in the pre-training, final stages. leading to ChatGPT.
He emphasized: “2010 was the age of measurement, now we are returning to the age of wonder and discovery. Many people are looking for the next new thing. Correcting the direction is more important than ever.”
Ilya Sutskever declined to provide further details on how the team addressed the problem, other than to say that SSI is working on an alternative method to scale its pre-training.
Behind the scenes, researchers in major AI labs have faced delays and disappointing results in the race to release a larger OpenAI language model beyond GPT-4 (two years ago). ).
“Training” large models costs tens of millions of dollars because hundreds of AI chips must be used at the same time.
Another problem is that large language models consume large amounts of data and have almost exhausted the available data in the world.
To overcome those challenges, researchers are exploring time-tested computing, a technique that improves existing AI models during the phase called “inference,” or when the model is in use.
This approach allows AI models to devote more processing power to tasks such as math problems, programming, or tasks that require human-like reasoning and decision-making abilities.
Speaking at TED AI, OpenAI researcher Noam Brown said: “Letting a bot think for just 20 seconds yields performance equivalent to calibrating a poker game model 100,000 times and training it 100,000 longer time”.
OpenAI has implemented this method in the large language model o1.
The o1 model can “think” about problems at many levels, similar to human thinking.
According to OpenAI, o1 outperforms other major language models for reasoning-intensive tasks in science, programming, and mathematics.
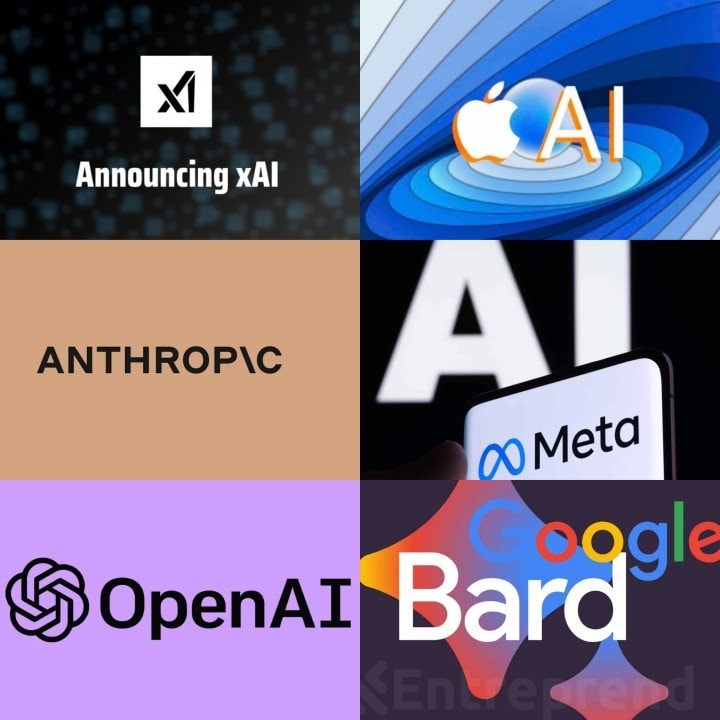
Researchers at other leading AI labs from Anthropic, xAI and Google DeepMind have been working to develop their own versions of the technology, according to five people familiar with the effort.
“We found that there are many simple things to do to quickly improve AI models,” he said at the inaugural technology conference in October. While everyone is catching up, we try to move forward in three steps.”
Google and xAI did not respond to Reuters’ inquiries, and Anthroponic did not immediately comment.
These effects could change the competitive landscape in the AI hardware market, which has seen strong demand for Nvidia’s AI chips.
Prominent venture capitalists from Sequoia to Andreessen Horowitz have poured billions of dollars into funding the development of expensive AI models at several labs, including OpenAI and xAI.
“This shift will take us from giant pre-trained clusters to reference clouds where servers are based,” said Sonya Huang, partner at prominent venture capital firm Sequoia Capital. in the cloud makes sense.
Demand for Nvidia’s AI chips, a cutting-edge technology, has pushed it past Apple to become the world’s most valuable company.
When asked about the potential impact on demand for its products, Navidi pointed to recent approaches to the engineering behind the o1 model.
Jenson Huang said at a conference in India in October: “We have found the second scaling law and that is the scaling law in testing… All these factors have led to the need Demand is extremely high for Blackwell.”